Automation's Hidden Menace
Robots and AI don't replace highly-skilled humans. But they block those people from training the next generation. Matt Beane's "The Skill Code" explains.
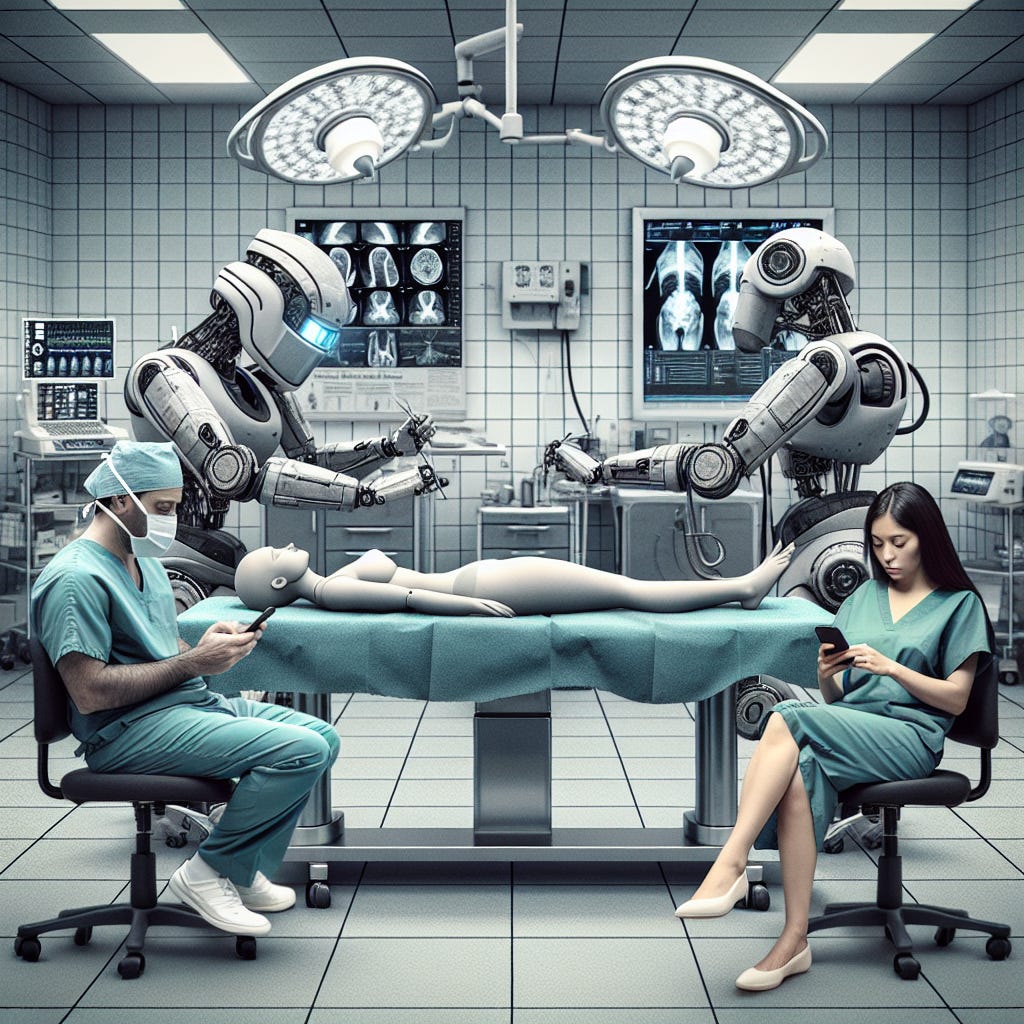
The other day at my annual eye checkup, I began with a young ophthalmologist, who measured the fluid pressure in my eyes, did some other checks, and had everything ready for my veteran doctor when he walked in.
As they talked over the data, he said, "Hmm, let me take his pressure again."
"See? It's actually a little lower than you wrote," he told his colleague. "The thing is, when you lift his eyelid a little for the machine, you're adding a very slight pressure from your finger. That can make the reading a ve…